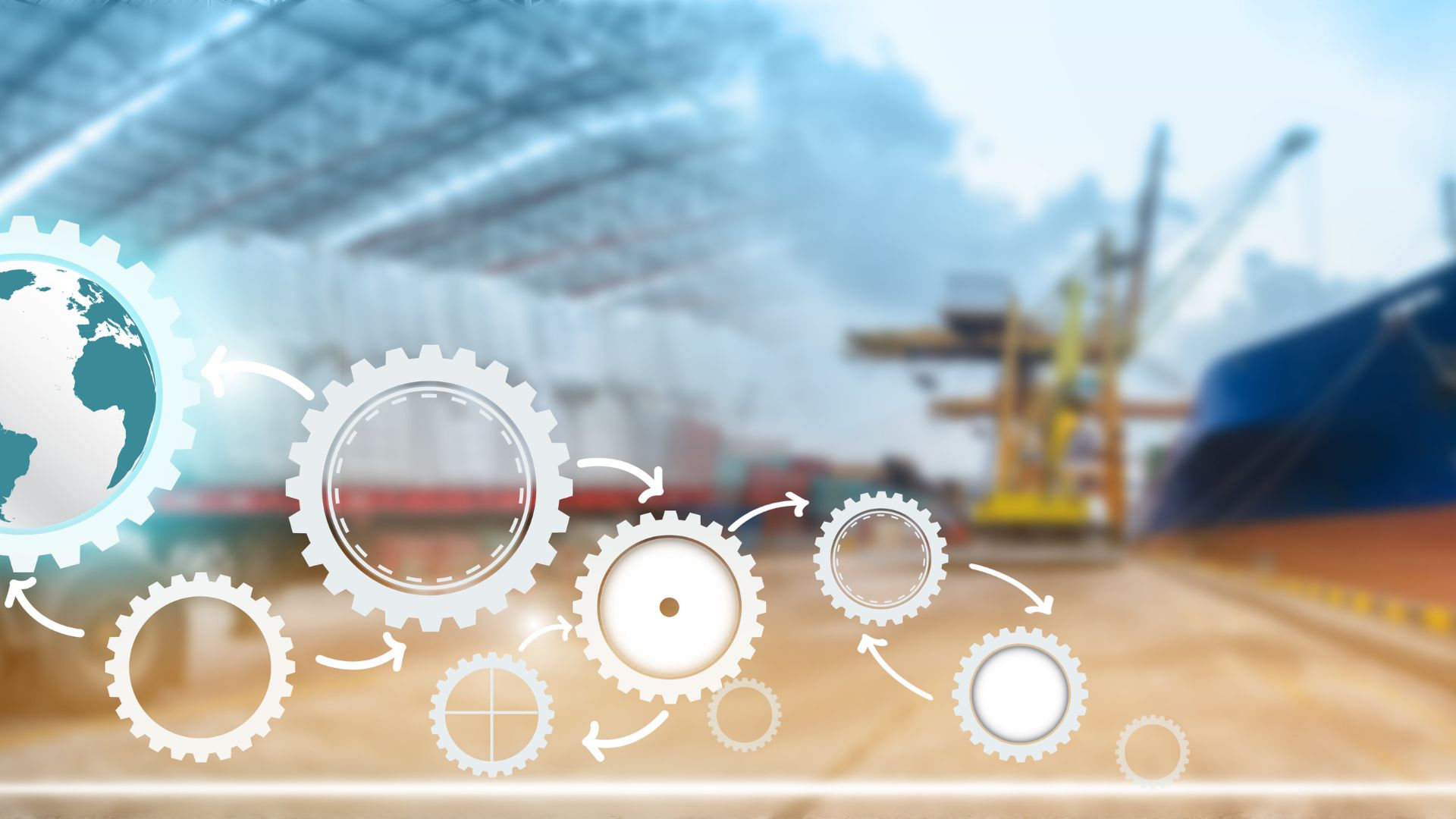
Industrial manufacturing has always been a proving ground for bleeding-edge technologies, fueling major leaps forward about every 10 to 20 years since the Industrial Revolution. Like it or not, AI seems to be the next catalyst that we’ll all have to contend with, which is exciting in both its potential and its uncertainty. Can it help with supply chain optimization?
We’d like to share how we are seeing AI manifest in industrial sourcing practices, pulling strictly from real-life examples in our own global manufacturing experience, and how buyers in this market may wish to further explore AI in their own projects. We promise that this is not a generic hype piece, but an informed commentary intending to cut through the chaff.
In the context of professionals working in industrial offshoring and supply chain optimization, AI can be boiled down to four toolsets:
- Text prediction: The most widespread use of AI today is by far that of generating written content (in many languages). Readers have likely heard the terms “large language model” and “generative AI,” together describing AI’s ability to produce lengthy, natural-sounding (albeit often genericized) text from minimal input prompts.
- Machine learning: Roughly speaking, machine learning is simply the use of mathematical algorithms that can automatically improve themselves through statistical feedback over time. Most industrial systems already include some form of machine learning technology, but when added to AI platforms, together this ongoing learning can be interacted with at a conversational level thanks to the large language model.
- Quantitative analysis: Computers have long been great at producing analytical information from input data sets, but humans have usually been required to interpret this analysis to find actionable insights. Now using AI’s text prediction components, AI can “read” computational output, and then provide conversational descriptions and observations from that data directly.
- Visualization: Most recently, AI development has grown a new branch that reaches into the visual domain. AI can directly generate graphs, charts, tables, renderings, illustrations, realistic photos and other visual works. (AI is also today producing convincing audio, video and augmented reality output, presenting increasing concerns about authenticity in all formats.)
From these four toolsets, readers can start to imagine ways that AI can be grafted onto supply chain optimization and management workflows – some good, some bad and some to be determined. Let’s look at specific example applications next.
AI in Supply Chain Optimization and Risk Mitigation
While not naming or recommending any particular AI services or vendors, we describe here a handful of AI capabilities available on the market today that are specifically geared toward optimizing and risk-managing industrial procurement applications.
- Pricing and cost analysis: If you’ve searched for flights online and have seen a message on the web page that says “Buy now, lowest price in three months,” it’s an example of contextual analysis of pricing data. Likewise, AI platforms can provide similar services in the form of pricing analysis for industrial goods, where input data is analyzed and then described in conversational ways to inform users of trends and conditions, as well as potential hazards and opportunities.
- Contract language: In industrial purchasing, contracts, terms and conditions, and similar legal language take time to generate, review and adopt. AI tools are helping companies speed through this legal language development process, generating text from legal-oriented language models and existing approved examples.
- RFP generation: In the past, companies often used document templates for their request for proposal (RFP) processes, working with standardized documents where they only filled in project-specific information. Now, AI tools can speed this process up further, populating templates faster from less input information, as well as generating missing text, submitting RFPs to vendors and even engaging vendors in text conversations.
- Supplier pre-qualification and vetting: AI’s ability to engage in digital conversations built from its language model is its main differentiator from other technologies, especially since the most advanced AIs can quite nearly mimic natural human responses. In this way, AIs can automate larger interview-like workflows, including supplier pre-qualification processes. AI can scour input datasets of potential vendors, match up vendor attributes to desired requirements, email and dialog with vendors to ask questions, and summarize results into a report for final human approval.
- Autonomous sourcing: If allowed, some AI systems can execute entire workflows within predetermined human constraints, such as sourcing and procurement transactions. Advanced systems essentially combine multiple functions, assigning risk factors between elements, and proceeding to contractual engagement if all parameters line up. This is increasingly common in MRO inventory management of low-risk products, such as with the automatic re-supply of gaskets and fasteners.
- Continuous process improvement: The machine learning component of AI can be implemented as a closed loop, specifically taking real-time data input and generating prospective future actions that would (in theory) improve these ongoing operations. AI-driven continuous process improvement is in a way no different than current industrial reliability improvement systems, but with the addition of the conversational interpretation and reporting function.
- Customer service: Since AI is inherently an interactive language system, it makes sense that customer service functions can be largely offloaded to AI chatbots, email respondents and soon, virtual verbal conversations.
- Graphical representation: As AI’s visualization abilities continue to grow, these platforms can increasingly produce compelling graphical representations of their written findings. Not only for data tables and charts, but for complex visualizations as well, such as logistic route efficiency comparisons, inventory heat maps, purchasing velocity trend curves, warehouse traffic pattern maps and overall supply chain optimization profiles.
Many Grains of Salt: Understanding AI’s Shortcomings
With all the promise, AI is still in its infancy, and so must be treated with cautious optimism – emphasis on cautious. We’ll briefly list the current main concerns that challenge AI below, and we advise buyers to keep both feet on the ground for the foreseeable future when entertaining AI tools in their supply chain optimization efforts.
- Hallucinations: Simply put, AI is well-known to fabricate incorrect information referred to as “hallucinations.” AI predicts text, and that text is compiled largely from uncontrolled access to public data and user interactions. There is no inherent method through which AI can ensure truth short of humans manually editing the data set, which can introduce more bias and inaccuracy as much as it can defend against it.
- Intellectual property protection: Information put into an AI tool serves to help train that tool, presenting the risk of exposing that data indirectly to the tool’s owners as well as other users. In this way, any company’s proprietary or commercially sensitive information can be unintentionally released. There is no guarantee of privacy with AI tools based on the nature of how they learn.
- Regulatory compliance: International trade businesses, transactions and entities have certain standards of care required of them. Factors such as data integrity and recordkeeping must be aligned with strict compliance requirements, in tandem with national security and trade laws as well. AI tools are a new animal in this forest of compliance, and may not natively meet these standards on their own.
- Generic or not application-specific: To reach mass marketability, emergent AI tools by their nature must be generic so that wider populations can contribute to their development. Popular text-generating AI tools are not domain experts in the same way that curated academic or scientific knowledgebases are. Even specialized AI tools just use domain-restricted data sets, and human moderators to parse them. None of this guarantees or even suggests final-verdict-grade expertise or sovereignty equivalent to consulting with a proven human expert.
Questions on Supply Chain Optimization?
Our experts at ITI Manufacturing are monitoring current AI tools and their role in supply chain optimization closely. If you have questions about manufacturing or supply chain logistics, we’d be happy to answer them. Contact us today.